The building blocks of all quantitative KPIs and performance measures are data elements..
Define them well enough so that KPIs do not become useless!
The body of data knowledge management is very complex and conceptual. It is a world best navigated by data management professionals. But that doesn’t mean that practitioners assessing performance should bury their heads in the sand and hope that someone else sorts out the data requirements of their KPIs (or performance measure).
If we don’t clearly specify the data elements our KPIs need, we end up implementing the wrong KPIs. Someone else will make assumptions about what KPIs mean, what data is available and, consequently, how it will be calculated.
We own the KPIs; we are responsible for how we implement them as intended.
Despite the complexity of the data management world, we can easily get to a point of articulating the data we need in a way that our data professionals can translate into technical requirements. To get to this point, we need a good understanding of four simple concepts:
1. Performance evaluation is obtained only from quantitative calculations.
2. Performance evaluation calculations are statistical summaries of raw data.
3. The raw data of a performance evaluation is described using data elements.
4. The data elements of the performance measure can be defined.
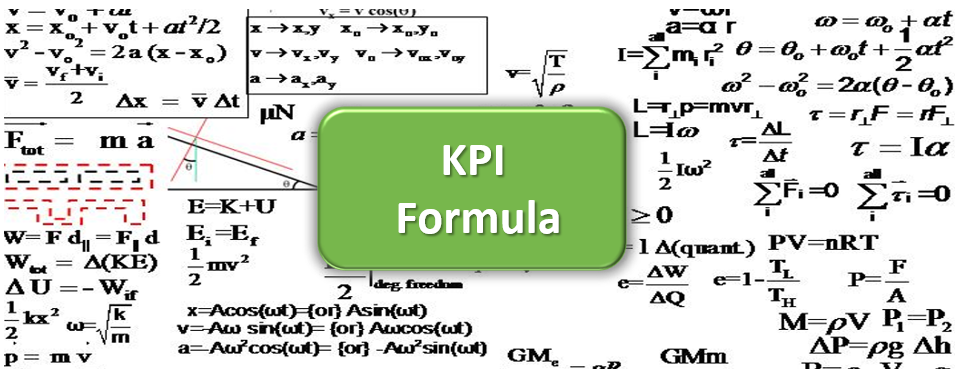
1. Performance evaluation is obtained only from quantitative calculations.
Performance appraisals are qualifications of direct evidence of a performance outcome that we want to monitor, at regular periods of time. To be quantitative, a performance appraisal needs a mathematical or statistical formula.
The Formula of a KPI describes how the raw data will be summarized into values for each time period. Raw data is what we collect in our business processes. For example, raw data can be:
- a customer’s satisfaction rating from the latest survey;
- the income obtained from a certain sale;
- the cost of last month’s electricity bill;
- the start date and end date of an employee’s term of office.
Each performance evaluation needs raw data for three parts of the calculation:
- the formula for calculating values such as customer satisfaction ratings;
- a period of time over which values are calculated, such as monthly;
- a field of application for segmenting or filtering the population to which the measure relates, such as active customers.
Sometimes the values of a performance measure are calculated based on one or more measures (such as how EBIT <Earnings Before Interest and Taxes> is calculated from revenue, cost of goods sold and operating expenses). But at its core, each performance metric is calculated by summarizing one or more different items of raw data.
2. Performance evaluation calculations are statistical summaries of raw data.
We synthesize raw data quantitatively using statistical processes, such as a number, a sum, a percentage or an average. Each performance measure has a frequency of calculation, which means that its values will be calculated in certain time periods, such as monthly or weekly.
Therefore, statistical processes will only apply to raw data collected during each specific time period. In order to calculate a monthly measure of recovery performance, only raw data on the amount of recovery carried out in that month shall be used for the calculation of that month.
Of course, it depends on the design of our measure which statistic we choose, the one that best answers the question the measure should answer. If we want, for example, to track how engaged our employees are, a percentage will not be nearly as useful as an average. Percentage tells us how much of our workforce has a certain level of engagement. But the average tells us how much engagement there is.
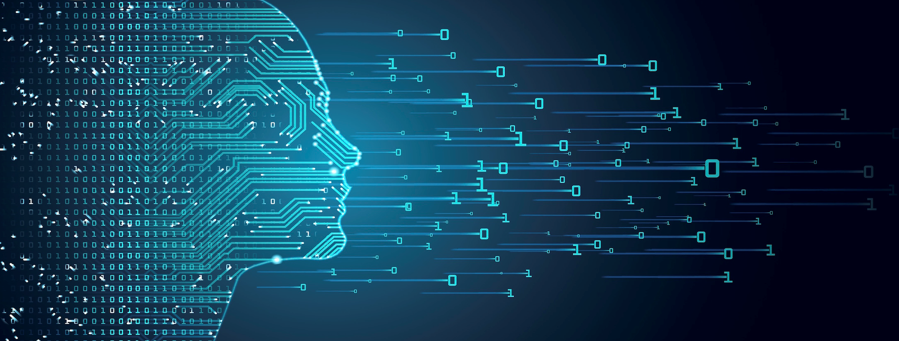
3. The raw data of a performance evaluation is described using data elements.
The raw data we synthesize for our performance evaluations comes from the repositories where the data is stored. No doubt every organization has a database where they store raw data about expenses, such as payment dates, amounts paid, types of expenses, names of suppliers, and so on.
Each raw data type is a field in a table in that database. In PuMP (performance measurement process), these fields are called “data elements”. For example:
- in a Customer Satisfaction table, there is a data field called “Overall satisfaction rating”;
- in a Sales table, there is a data field called “Amount received”;
- in an Expenditure table, there is a data field called “Amount paid”, a data field called “Expenditure type” and so on;
- in an Employee Engagement table, there is a data field called “Start Date” and a field called “End Date”.
When defining data requirements for a performance evaluation, we need to know the correct field name and table name. For a performance assessment called Electricity Expenditure, calculated monthly, the calculation can be written as follows:
Amount (Amount paid), after (Date of payment) formatted as “mm/ yyyy”, where (Type of expenditure) = “Electricity”
There will be several ways to write the formula of any assessment. This is not a problem, as long as we write it clearly enough that anyone can understand what we are trying to achieve.
It is also important that as we begin to implement our assessments, we understand a little more about the specifics of how our data elements are or should be defined. This matters in two situations:
- when we need to find the best data for an evaluation;
- when we need to create new data for an evaluation.
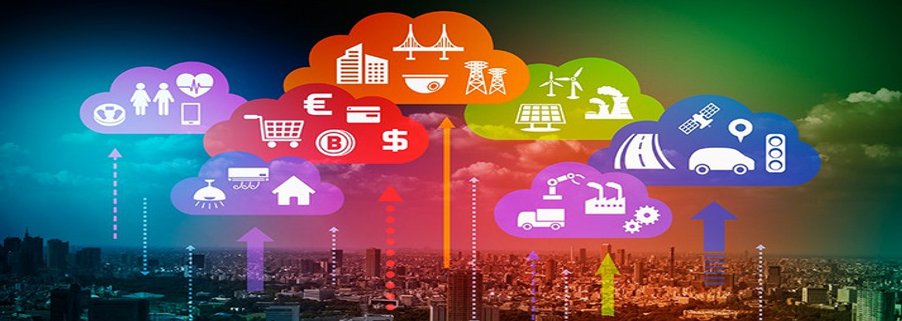
4. The data elements of the performance measure can be defined.
Imagine if we discovered that the Delivery Cycle Time measure was calculated from data based on days, but we wanted to capture changes in the measure in just a few hours. Or our Customer Lifetime Spend measure was calculated based on data where a lot of customers have several different ID codes that are counted as if they were separate customers.
These problems stem from data elements that are not configured to meet the requirements of our measures. For this reason, it helps to pay attention to five specific parts of a data element definition:
- Name – so our measure definition refers to the correct data element.
- Description – to make sure that the data item is what we think it is. For example, “Amount paid” may be different from “Invoiced amount” because it is the invoice amount minus any credit that may have existed.
- Format – to make sure the data units match the units provided for our measure. For example, we may need “Amount Paid” to be in Australian dollars, “Activity Start Date” to be a date-hour value such as yy-mm-dd, and “Overall Satisfaction Rating” to be an integer.
- Ranges of valid values – to make it easier to identify data errors and fix them before calculating our measurement values. For example, “Overall satisfaction rating” may only take values from 0 to 10, and “End date” may not be a value in the future.
- Standardization – so that it is possible to link data from datasets or tables or databases, where our measure is calculated from several data elements collected in different places.
These five things we mentioned above are things that need to be discussed with data management professionals. Only through these conversations – merging business requirements and technical requirements – can we be sure that the right measures are implemented in the right ways.
Data elements are the building blocks of KPIs. If we fail to define them clearly, KPIs fall apart.
Article source: www.measureupblog.com
For specific and specialized solutions from QQinfo, please visit this page: QQsolutions.
In order to be in touch with the latest news in the field, unique solutions explained, but also with our personal perspectives regarding the world of management, data and analytics, we recommend the QQblog !