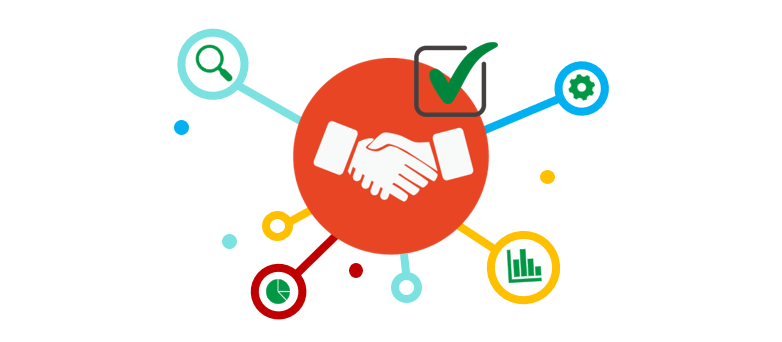
The principle of “Garbage In, Garbage Out” emphasizes a key truth: the quality of input directly determines the quality of output. As organizations aim to harness the value of vast volumes of data, managing data quality centrally becomes increasingly complex. Many organizations are discovering that decentralizing data quality to domain experts allows them to profile, validate, and curate data into reliable products, fostering greater business confidence and use.
Data Quality and Trust: The Business Impact
The quality and trustworthiness of data significantly influence how and whether it is used, impacting various aspects of a business, including:
- Decision-making
- Compliance
- Customer satisfaction
- Market efficiency
- Competitive advantage
- Overall business growth
For example, a retail company found that its loyalty program failed to identify duplicate customer records, resulting in a 30% increase in marketing costs due to duplication. This also led to customer frustration, as they received conflicting information and promotions.
Maintaining high standards for data quality and trust is crucial for building a reliable and successful business. By ensuring this data is made available through a data marketplace, organizations can offer consumers a trusted, single version of the truth.
Data Quality: Accuracy and Reliability
Accurate, high-quality data is essential for making informed decisions. When data is inaccurate or unreliable, it can lead to poor decision-making, damaging the credibility and success of both the data product** and the data marketplace.
For instance, a supply chain company suffered significant losses due to outdated and inconsistent inventory data. This resulted in accepting orders that couldn’t be fulfilled on time, leading to contract breaches, financial penalties, and a loss of customer trust, ultimately costing the company millions in long-term contracts.
The value of data products in a marketplace is directly linked to their quality levels for a domain-centric use-case. High-quality data products are more likely to deliver value and meet consumer needs, strengthening the marketplace’s reputation.
**A data product is like a cake, created from ingredients like raw data, algorithms, and analytics. The result is a fully “baked” product, ready to deliver value—whether as insights, dashboards, or predictions. Unlike raw data, it’s complete and easy to use.
Trust: User Confidence in the data
Consumers need to trust that the data they are purchasing or accessing is accurate, reliable, and suitable for their needs. Without trust, consumption decreases and there is no incentive for the data producers to produce these data products, hindering overall data marketplace growth. Sellers need to ensure that their data products are of high quality to build a positive reputation. Consistent delivery of high-quality data helps establish credibility and fosters long-term relationships with buyers.
A best-in-class organization achieves consistent delivery of high-quality data products by combining clear objectives, strong data governance, and robust development processes. They define standards for data quality, adopt agile methodologies, and utilize scalable technology to ensure reliability and adaptability. Multidisciplinary teams collaborate to build products tailored to user needs, while continuous feedback and performance monitoring drive improvement. By fostering a culture of quality and investing in skilled teams and modern tools, they create data products that are accurate, user-friendly, and impactful.
As an example, an e-commerce platform allowed sellers to post product reviews without verifying their authenticity, leading to a loss of customer trust due to fabricated or exaggerated ratings. This resulted in declining sales, reputational damage, and increased regulatory scrutiny, forcing the company to make significant investments to overhaul its systems, policies, and practices.
A company would need to invest in review verification tools, identity checks, and moderation systems to ensure authenticity. It must implement stricter policies, comply with regulations, and educate users on ethical practices. Additional efforts include hiring moderators, rebuilding trust with PR campaigns, and using analytics to improve review quality. These investments aim to restore trust and reputation.
Exemple și cazuri de utilizare
The following examples and use cases explore the business impact of data quality and trust.
Regulatory Compliance
As shown in the example above, poor quality and untrusted data can lead to regulatory scrutiny. Adherence to data quality standards and regulations (such as GDPR, CCPA) is necessary to ensure compliance. Non-compliance can lead to legal issues, fines, and damage to the marketplace’s reputation.
For example, a pharmaceutical company in a highly regulated market faced severe consequences due to poor data management. Inaccurate and incomplete reporting led to hefty fines, delayed drug approvals, and temporary production halts, causing significant revenue loss and allowing competitors to gain an edge. The company also suffered reputational damage and was placed under stricter regulatory scrutiny, forcing costly investments in compliance and data governance systems.
Ensuring data quality often involves implementing robust data security measures to protect sensitive information and maintain data integrity.
Customer Satisfaction
Customers expect data products to meet certain quality standards. If data products are unreliable or inaccurate, customer satisfaction will decrease, leading to negative reviews and potential loss of business.
At a financial services firm, employees were dissatisfied with the data quality available through the new analytics platform, which was inconsistent and inaccurate. This led to increased manual work, reduced productivity, and low adoption of the platform, causing delays in decision-making, frustrated clients, and higher turnover rates. The company faced significant costs in recruiting and training new staff to replace those who left.
High-quality data products reduce the need for extensive support and troubleshooting, leading to better overall customer experiences.
Market Efficiency
High-quality data allows for more accurate matching between buyers and sellers, enhancing the efficiency of transactions in the marketplace.
As an example, an online marketplace for data products used advanced algorithms to accurately match buyers with relevant sellers, significantly improving efficiency. Buyers saved time by being directed to the most appropriate data products, while sellers increased transaction volume by reaching the right customers quickly. The matching system encouraged high-quality data offerings, reduced transaction costs, and facilitated better decision-making for buyers, ultimately leading to increased business outcomes for both parties.
Quality data is easier to integrate and use across different systems and platforms, increasing the utility and effectiveness of data products built from it.
Competitive Advantage
A high-quality and trusted data marketplace generates competitive advantages for data product consumers by providing access to reliable, accurate, and up-to-date data that enhances decision-making, innovation, and operational efficiency.
As an example, a retail company that invested in high-quality, trusted customer data gained a significant competitive advantage by offering personalized shopping experiences. By leveraging accurate insights into customer preferences and behaviors, the company was able to tailor promotions, recommend products, and optimize inventory in real-time, leading to increased customer satisfaction and loyalty. By feeding this high-quality, curated data into an AI/ML model, the business was able to closely predict trends, respond faster to dynamic market demands, and outpace competitors who were using less reliable data, ultimately driving higher sales and market share.
Reliable data enables innovation and the development of new data products and services, driving growth and advancement.
Scalability and Growth
Ensuring data quality supports the scalability of the marketplace by maintaining consistency and reliability as the volume of transactions and participants grows.
As an example, in a large multinational corporation, different departments acted as both buyers and sellers of data. For example, the marketing team sold customer insights to the product development team, while finance shared budget and performance data with both marketing and operations. This internal data marketplace improved collaboration, ensured efficient data sharing, and enabled faster, data-driven decision-making, leading to optimized resources and a more agile business model.
A focus on quality helps to build a strong, trustworthy brand, which is essential for attracting new users and expanding the marketplace.
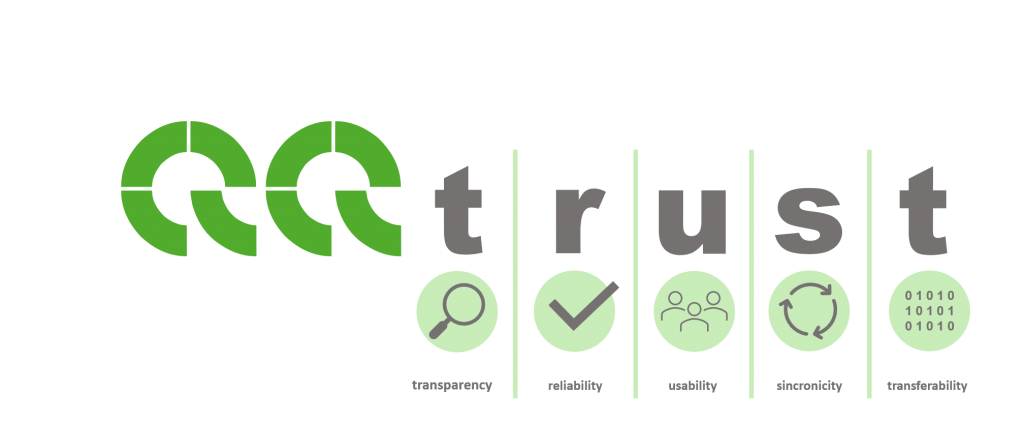
In context of data trust, QQinfo has created a toolkit, methodologies, and best practices that minimize the risks of using erroneous analytics in decision-making.
No matter how fast, innovative, interactive, or efficient a BI system is, the base-point in the construction of a set of analytics must be the CORRECTNESS OF THE INFORMATION PROVIDED.
This correctness is determined by 2 essential factors:
- The data used should be accurate, complete, and fresh enough;
- All data processing (intermediate and final) does not alter the quality of the source information.
QQtrust™ is a set of methods and solutions that QQinfo applies to the analytics and solutions provided to maximize confidence in the correctness of the information delivered as a decision support system and to minimize associated risks. To this end, based on more than 15 years of experience, we have added to this portfolio specific solutions for each type of risk identified.
The technology has multiple benefits, but it also comes with a flip side of the coin, which requires a specific approach to minimize its risks. QQtrust™ is a conclusive example of this.
Click here for details about QQtrust™.
Conclusion
Data quality and trust are foundational to the success of any data product marketplace. By ensuring high-quality, reliable data, businesses can enhance decision-making, maintain compliance, improve customer satisfaction, and gain a competitive edge. As the marketplace scales, maintaining data integrity ensures continued growth and long-term success.
Article sourced here: https://community.qlik.com/ and enriched by QQinfo.
For information about Qlik™, click here: qlik.com.
For specific and specialized solutions from QQinfo, click here: QQsolutions.
In order to be in touch with the latest news in the field, unique solutions explained, but also with our personal perspectives regarding the world of management, data and analytics, click here: QQblog !